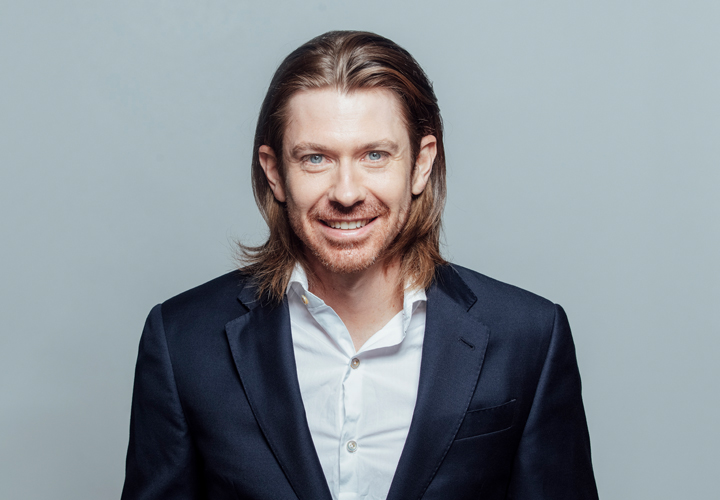
John Hayes is CEO and founder of autonomous vehicle software innovator Ghost Autonomy.
Prior to Ghost, John founded Pure Storage, taking the company public (PSTG, $11 billion market cap) in 2015. As Pure’s chief architect, he harnessed the consumer industry’s transition to flash storage (including the iPhone and MacBook Air) to reimagine the enterprise data center inventing blazing fast flash storage solutions now run by the world’s largest cloud and ecommerce providers, financial and healthcare institutions, science and research organizations and governments.
Like Pure, Ghost uses software to achieve near-perfect reliability and re-defines simplicity and efficiency with commodity consumer hardware. Ghost is headquartered in Mountain View with additional offices in Detroit, Dallas and Sydney. Investors including Mike Speiser at Sutter Hill Ventures, Keith Rabois at Founders Fund and Vinod Khosla at Khosla Ventures have invested $200 million in the company.
Now, let’s get into it, shall we?
M.R. Rangaswami: How does the expansion of LLMs to new multi-modal capabilities extend their application to new use cases?
John Hayes: Multi-modal large language models (MLLMs) can process, understand and draw conclusions from diverse inputs like video, images and sounds, expanding beyond simple text inputs and opening up an entirely new set of use cases from everything from medicine to legal to retail applications. Training GPT models on more and more application specific data will help improve them for their specific task. Fine-tuning will increase the quality of results, reduce the chances of hallucinations and provide usable, well-structured outputs.
Specifically in the autonomous vehicle space, MLLMs have the potential power to reason about driving scenes holistically, combining perception and planning to generate deeper scene understanding and turn it into safe maneuver suggestions. The models offer a new way to add reasoning to navigate complex scenes or those never seen before.
For example, construction zones have unusual components that can be difficult for simpler AI models to navigate — temporary lanes, people holding signs that change and complex negotiation with other road users. LLMs have shown to be able to process all of these variables in concert with human-like levels of reasoning.
M.R.: How is this new expansion impacting autonomous driving, and what does it mean for the “autonomy stack” developed over the past 20 years?
John: I believe MLLMs present the opportunity to rethink the autonomy stack holistically. Today’s self-driving technologies have a fragility problem, struggling with the long tail of rare and unusual events. These systems are built “bottoms-up,” comprised of a combination of point AI networks and hand-written driving software logic to perform the various tasks of perception, sensor fusion, drive planning and drive execution – all atop a complicated stack of sensors, maps and compute.
This approach has led to an intractable “long tail” problem – where every unique situation discovered on the road requires a new special purpose model and software integration, which only makes the total system more complex and fragile. With the current autonomous systems, when the scene becomes overly complex to the point that the in-car AI can no longer safely drive, the car must “fall-back” – either to remote drivers in a call center or by alerting the in-car driver.
MLLMs present the opportunity to solve these issues with a “top-down” approach by using a model that is broadly trained on the world’s knowledge and then optimized to execute the driving task. This adds complex reasoning without adding software complexity – one large model simply adds the right driving logic to the existing system for thousands (or millions) of edge cases.
There are challenges implementing this type of system today, as the current MLLMs are too large to run on embedded in-car processors. One solution is a hybrid architecture, where the large-scale MLLMs running in the cloud collaborate with specially trained models running in-car, splitting the autonomy task and the long-term versus short-term planning between car and cloud.
M.R.: What’s the biggest hurdle to overcome in bringing these new, powerful forms of AI into our everyday lives?
John: For many use cases, the current performance of these models is already there for broad commercialization. However, some of the most important use cases for AI – from medicine to legal work to autonomous driving – have an extremely high bar for commercial acceptance. In short, your calendar can be wrong, but your driver or doctor can not.
We need significant improvements on reliability and performance (especially speed) to realize the full potential of this technology. This is exactly why there is a market for application-specific companies doing research and development on these general models. Making them work quickly and reliably for specific applications takes a lot of domain-specific training data and expertise.
Fine-tuning models for specific applications has already proven to work well in the text-based LLMs, and I expect this exact same thing will happen with MLLMs. I think companies like Ghost, who have lots of training data and a deep understanding of the application, will dramatically improve upon the existing general models. The general models themselves will also improve over time.
What is most exciting about this field is the trajectory — the amount of investment and rate of improvement is astonishing — we are going to see some incredible advances in the coming months.
M.R. Rangaswami is the Co-Founder of Sandhill.com